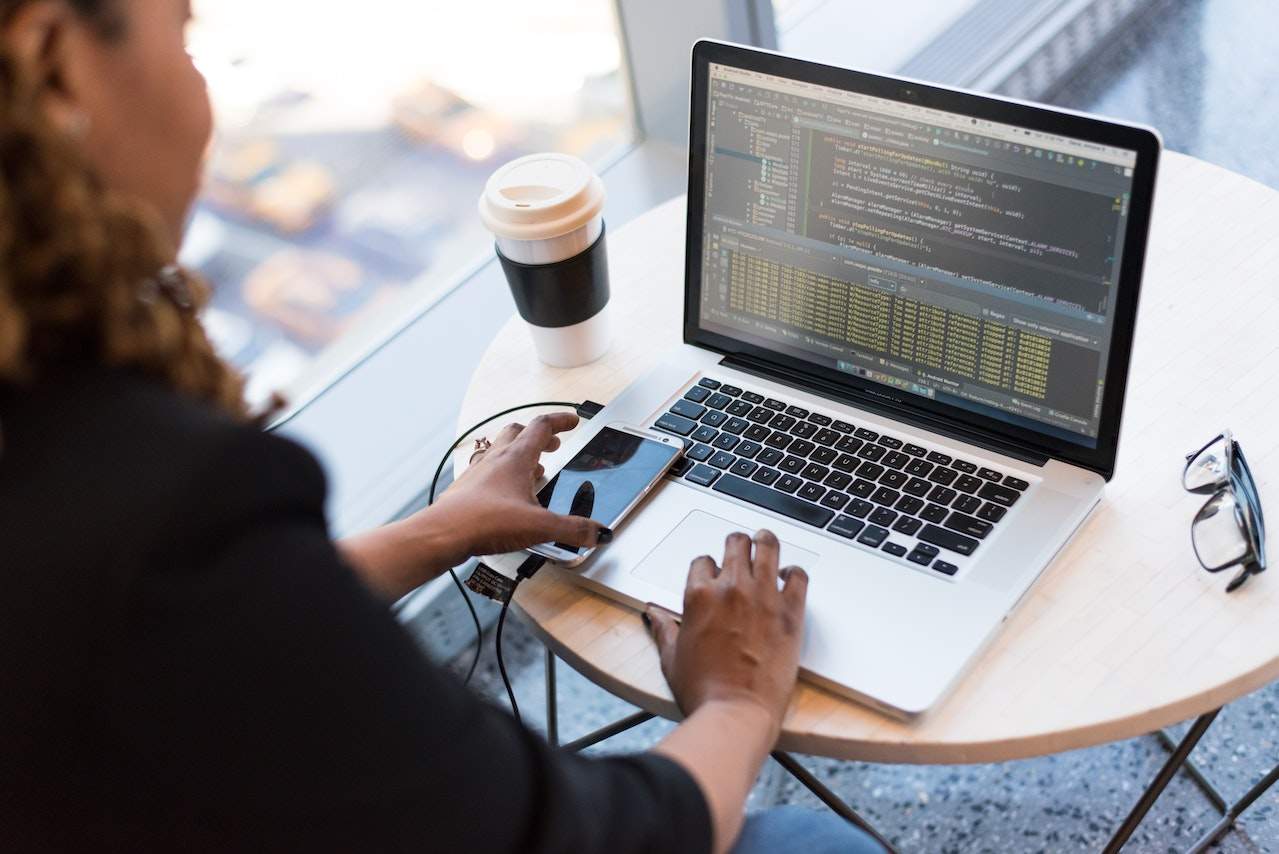
Building Smarter Data Systems: Top 5 Data Engineering Trends to Watch Out for in 2023
Data engineering is an ever-evolving field that requires constant adaptation to stay ahead of the curve. With the world becoming increasingly data-driven, businesses are looking for ways to build smarter and more efficient data systems that can handle large amounts of information and provide actionable insights.
In 2023, we can expect to see some exciting trends emerge in the world of data engineering that will transform the way we work with data. From the rise of cloud-native data engineering to the integration of machine learning and artificial intelligence, these trends are set to revolutionize the way we approach data management.
In this article, we’ll explore the top 5 data engineering trends to watch out for in 2023 and how utilising data engineering services will impact the future of businesses. So, if you’re interested in building smarter data systems that can help your business stay ahead of the competition, read on to find out more!
Trend 1: The Rise of Machine Learning and AI in Data Engineering
The integration of machine learning and artificial intelligence (AI) is set to be one of the most significant trends in data engineering in 2023. Machine learning and AI algorithms can help automate data processing and analytics, making data engineering more efficient and accurate. With machine learning and AI, data engineers can identify patterns and insights that would be difficult or impossible to detect manually. Additionally, machine learning and AI can help improve data quality by detecting and correcting errors in real-time.
One example of how machine learning and AI can be used in data engineering is in predictive maintenance. Predictive maintenance uses machine learning algorithms to analyze data from sensors and predict when equipment is likely to fail. By predicting equipment failures before they happen, businesses can save money by avoiding costly downtime and repairs. Machine learning and AI can also be used to automate data cleaning and normalization, making data engineering more efficient and accurate.
Despite the benefits of machine learning and AI, there are also some challenges to consider. One of the biggest challenges is the need for high-quality data. Machine learning and AI algorithms rely on large amounts of data to learn and improve, so businesses need to ensure they have access to high-quality data to make the most of these technologies. Additionally, machine learning and AI algorithms can be complex and difficult to implement, so businesses need to have the right expertise in place to make the most of these technologies.
Trend 2: Cloud-Based Data Engineering Solutions
Cloud-based data engineering solutions are becoming increasingly popular, and we can expect this trend to continue in 2023. Cloud-based data engineering offers a number of benefits, including scalability, flexibility, and cost-effectiveness. With cloud-based data engineering solutions, businesses can scale their data processing and analytics capabilities up or down as needed, without having to invest in additional hardware or software. Additionally, cloud-based solutions can be more cost-effective than on-premise solutions, as businesses only pay for what they use.
One example of a cloud-based data engineering solution is Amazon Web Services (AWS), which offers a range of tools and services for data processing and analytics. AWS offers services like Amazon S3 for data storage, Amazon Redshift for data warehousing, and Amazon EMR for data processing and analytics. Other cloud providers like Microsoft Azure and Google Cloud Platform also offer similar services.
There are some risks to consider with cloud-based data engineering solutions. One of the biggest risks is the security of data. Businesses need to ensure that their data is secure when using cloud-based solutions, especially if they are using public cloud providers. Additionally, businesses need to be aware of the potential for vendor lock-in when using cloud-based solutions. If a business relies heavily on a particular cloud provider, it can be difficult and expensive to switch to a different provider in the future.
Trend 3: The Importance of Real-Time Data Processing
Real-time data processing is becoming increasingly important, especially in industries like finance, healthcare, and manufacturing. Real-time data processing enables businesses to make decisions quickly based on up-to-date information, which can be critical in fast-moving industries. Real-time data processing can also help businesses detect and respond to issues as they happen, rather than after the fact.
One example of real-time data processing is in fraud detection. Financial institutions can use real-time data processing to detect and prevent fraudulent transactions as they happen. Real-time data processing can also be used in healthcare to monitor patients in real-time and detect potential issues before they become more serious.
There are some challenges to consider with real-time data processing. One of the biggest challenges is the need for high-performance computing and storage capabilities. Real-time data processing requires fast processing and analysis of data, which can be resource-intensive. Additionally, businesses need to ensure that their data is of high quality and relevant for real-time processing.
Trend 4: The Emergence of Data Mesh Architecture
Data mesh architecture is a new approach to data engineering that is gaining popularity in 2023. Data mesh architecture is based on the idea of decentralizing data ownership and management, and empowering individual teams to manage their own data. In data mesh architecture, data is treated as a product, and individual teams are responsible for managing and maintaining their own data products.
One of the benefits of data mesh architecture is that it can help improve data quality by empowering individual teams to take ownership of their own data. Additionally, data mesh architecture can help improve collaboration between teams and reduce the risk of data silos. By decentralizing data ownership, businesses can also reduce the risk of data breaches or other security issues.
There are some challenges to consider with data mesh architecture. One of the biggest challenges is the need for a significant cultural shift within organizations. Data mesh architecture requires a high degree of collaboration and communication between teams, which can be difficult to achieve in some organizations. Additionally, data mesh architecture can be complex to implement and requires a significant investment in technology and expertise.
Trend 5: The Rise of Low-Code and No-Code Data Engineering Tools
Low-code and no-code data engineering tools are becoming increasingly popular, especially among business users who may not have a technical background. Low-code and no-code tools enable users to build data pipelines and workflows without having to write code. This can help democratize data engineering and enable more people within organizations to work with data.
One example of a low-code data engineering tool is Microsoft Power Platform, which enables users to build data pipelines and workflows using a drag-and-drop interface. Other examples include Google Cloud Dataprep and AWS Glue. No-code tools like Tableau and Power BI can also enable users to analyze and visualize data without having to write code.
There are some risks to consider with low-code and no-code data engineering tools. One of the biggest risks is the potential for data quality issues. Without the proper expertise, users may not be aware of best practices for data engineering, which can lead to errors or inconsistencies in data. Additionally, low-code and no-code tools may not be suitable for all use cases, especially for more complex data engineering tasks.
Challenges and Risks to Consider
While these trends offer exciting opportunities for businesses looking to build smarter data systems, there are also some challenges and risks to consider. Businesses need to be aware of these challenges and risks in order to make the most of these trends.
One of the biggest challenges in data engineering is the need for high-quality data. Without high-quality data, businesses will struggle to make accurate decisions and gain insights from their data. Additionally, businesses need to ensure that their data is secure, especially if using cloud-based solutions.
Another challenge is the need for expertise in data engineering and analytics. While low-code and no-code tools can help democratize data engineering, businesses still need to have the right expertise in place to make the most of these tools. Additionally, businesses need to be aware of the potential for data breaches or other security issues, especially when working with sensitive data.
Conclusion: The Future of Data Engineering
Overall, data engineering is an exciting field that offers many opportunities for businesses to build smarter data systems. In 2023, we can expect to see some exciting trends emerge, including the integration of machine learning and AI, cloud-based data engineering solutions, real-time data processing, data mesh architecture, and low-code and no-code data engineering tools. While these trends offer many benefits, businesses need to be aware of the challenges and risks involved and ensure they have the right expertise and processes in place to make the most of these trends. With the help of a leading data engineering company, businesses can build smarter data systems that enable them to stay ahead of the competition and make better decisions based on data-driven insights.
__________________________________________________________________________
Author Bio:
Ulaganathan is a digital marketing specialist at Indium Software, a leading data engineering company that specializes in implementing robust data solutions for 100+ global clients. With in-hand experience in the field, has worked with a diverse range of clients across multiple industries to enhance brand awareness and drive lead generation.